When asked to think of AI, most people are likely to think of usual suspects like chatbots, voice assistants such as Google Assistant, Siri, or Alexa. And ofcourse, who wouldn’t think of the recent big names like ChatGPT! While all of these are spot-on examples of AI, they primarily fall under two AI categories: Conversational AI and Generative AI.
But what’s the real essence behind the terms “conversational” and “generative”? How do they stand apart, and why should businesses care? In this blog, we’ll answer these questions and provide you with easy to understand examples of how your enterprise can leverage these technologies to stay ahead of the competition.
Let’s dive in with the definitions.
What is Conversational AI?
In the vast realm of AI, Conversational AI stands as a bridge between machines and humans, facilitating interactions that mirror natural human conversations. With the ability to understand intent and piecing together necessary information, they ensure the right resolution to customer’s queries, and fast!
Designed to help machines understand, process, and respond to human language in an intuitive and engaging manner.
Conversational AI thrives on a blend of advanced technologies, ensuring bots not only grasp human language but also respond with human-like precision. The 4 pillars of Conversational AI are:
Machine Learning (ML)
At the heart of Conversational AI, ML employs intricate algorithms to discern patterns from vast data sets. This continuous learning enhances the bot’s understanding and response mechanism. For instance, ML powers image recognition, speech recognition, and even self-driving cars, showcasing its versatility across sectors.
Natural Language Processing (NLP)
NLP transforms raw user input into machine-readable data, ensuring bots comprehend and generate relevant responses. It encompasses:
- Lexical Analysis: Segmenting text into words or phrases.
- Syntax and Semantic Analysis: Deciphering word relationships and text meanings.
- Sentiment Analysis: Gauging the emotional tone of a text.
- Output Transformation: Crafting human-like responses based on input.
Data Mining
Beyond mere pattern recognition, data mining extracts valuable insights from conversational data. It aids in refining the AI system’s functionality. For instance, by analyzing customer behaviors, AI can segment customers, enabling businesses to tailor their marketing strategies.
Automatic Speech Recognition (ASR)
Essential for voice interactions, ASR deciphers human voice inputs, filters background disturbances, and translates speech to text. Tools like voice-to-text dictation exemplify ASR’s capability to streamline tasks.
Together, these components forge a Conversational AI engine that evolves with each interaction, promising enhanced user experiences and fostering business growth.
Must read: 10 Top conversational AI platforms in 2024
What is Generative AI?
Venturing into the imaginative side of AI, Generative AI is the creative powerhouse in the AI domain. Unlike traditional AI systems that rely on predefined rules, it uses vast amounts of data to generate original and innovative outputs. By analyzing patterns and learning from existing examples, generative AI models can create realistic images, music, text, and more, often surpassing human imagination.
Employs algorithms to autonomously create content, such as text, images, music, and more, by learning patterns from existing data.
The backbone of generative AI is the Generative Adversarial Network (GAN).
Generative Adversarial Networks (GANs)
Generative Adversarial Networks (GANs) are a class of generative AI models that have revolutionized the field of artificial intelligence by generating realistic and high-quality content. GANs consist of two parts: a generator, which creates images, and a discriminator, which evaluates them. The two work in tandem, effectively “teaching” each other to produce better results. This adversarial process leads to the generation of highly realistic content.
Variational Autoencoders (VAEs)
Variational Autoencoders (VAEs) are a type of generative AI model that combine concepts from both autoencoders and probabilistic modeling. They are powerful tools for learning representations of complex data and generating new samples. VAEs allow for the creation of new instances that can be similar to your input data, making them great for tasks like image denoising or inpainting.
Related read: 9 Benefits of Generative AI in Enterprises
Having understood the basics and their applications, let’s explore how the two technologies differ in the next section.
Main differences between Conversational AI and Generative AI
Simply put, Conversational AI focuses on human-like conversations while Generative AI focuses on creating or “generating” new content in different forms. The following table highlights the main differences:
Conversational AI | Generative AI | |
Objective | Facilitates interactive dialogues between humans and AI, emphasizing natural language processing. | Concentrates on generating fresh and innovative content without explicit instructions. |
Inputs & outputs | Takes the user’s natural language as input and returns context-sensitive responses. | Processes various data types to craft new content based on learned patterns. |
Training & learning | Primarily trained using vast conversation datasets, ensuring the model’s ability to emulate human-like interactions. | Utilizes diverse data, from text to images, to identify patterns and produce content accordingly. |
Applications & use-case | Commonly employed in virtual assistants, chatbots, customer support, language translation tools, and voice-controlled interfaces. | Widely applied in realms like virtual reality, gaming, artistic creations, content generation, product innovations, and multimedia enhancements. |
Interactivity | High engagement potential, adapting responses based on real-time user interactions, questions, and feedback. | Generally provides content with minimal interaction, relying heavily on its training data for content generation. |
Complexity | Capable of comprehending and reacting to intricate user inquiries, ideal for real-world applications such as customer service. | Generates detailed and imaginative outputs, though might not always grasp the context or specific user inclinations. |
Feedback loop | Benefits from user feedback, enabling iterative model enhancements. | Often refines outputs based on curated data, but might lack direct user feedback mechanisms. |
Dependencies | Anchored by high-quality, diverse conversational data to ensure relevance. | Dependent on a wide range of data types to hone its content generation capabilities. |
Latency | Engineered for real-time responses, prioritizing prompt user interactions. | Might require more processing time, especially for intricate content generation. |
Scalability | Adapts well to increasing user interactions, ensuring consistent performance. | Scales effectively with data, refining the richness of generated content over time. |
Versatility | Best suited for applications demanding real-time interactions and feedback. | Exhibits adaptability across a spectrum of domains needing content creation. |
Data sensitivity | Requires consistent data quality; anomalies can lead to response inaccuracies. | Resilient to varied data inputs but can deviate in output quality with skewed training data. |
Limitations | Might occasionally struggle with highly technical or out-of-context queries. | While innovative, can occasionally produce outputs that lack contextual relevance. |
Real-life applications of Conversational AI
Conversational AI’s ability to provide instant, accurate, and personalized responses makes it an invaluable tool for businesses across industries aiming to enhance customer satisfaction and drive growth. Here are a few applications:
- Customer support chatbots: These AI-driven chatbots are designed to handle a wide range of customer queries, from simple FAQs to more complex issues. They can operate 24/7, ensuring that customer concerns are addressed promptly.
- Voice assistants: Platforms like Alexa, Google Assistant, and Siri use conversational AI to understand and respond to user commands. They can set reminders, play music, provide weather updates, and more.
- Social media interactions: With the rise of social media platforms, businesses are leveraging conversational AI to interact with customers on platforms like Instagram, Facebook Messenger, and WhatsApp. These AI tools can answer product-related questions, provide recommendations, and even facilitate purchases.
- Virtual agents for contact centers: Conversational AI can streamline the operations of contact centers by handling routine inquiries, thereby allowing human agents to focus on more complex issues.
- Personalized marketing: By analyzing user interactions, conversational AI can provide personalized product recommendations and promotional offers, enhancing the shopping experience for users. For example, Yellow.ai’s Engage helps retailers craft Generative AI powered digital campaigns that increase revenue per campaign by 15% and boost lead generation by 20%.
Real-life applications of Generative AI
Learn how Generative AI is being used to boost sales, improve customer service, and automate tasks in industries such as BFSI, retail, automation, utilities, and hospitality.
- Content creation: Generative AI can produce a variety of content, from text (ChatGPT, Google Bard) and images (DALL-E, Midjourney) to music (Musenet) and videos (Runway). This is particularly useful for creative industries, where AI can assist in generating initial drafts or ideas.
- Enhanced customer interactions: Generative AI can improve customer interactions through enhanced chat and search experiences. It can provide more human-like responses and even generate content based on user preferences.
- Data exploration: Generative AI can explore vast amounts of unstructured data, providing insights through conversational interfaces and summarizations. This is especially beneficial for businesses looking to derive actionable insights from their data.
- Repetitive tasks automation: Tasks like replying to requests for proposals (RFPs), localizing marketing content in multiple languages, and checking customer contracts for compliance can be automated using generative AI.
- Innovations in various fields: Generative AI has found applications in drug and chip design, material science development, and more. Its ability to generate novel solutions based on existing data makes it a valuable tool for research and development.
The future of AI: Leveraging Conversational AI and Generative AI for enterprises
The future of enterprise interactions does not lie in the “VERSUS” but is in the “AND” i.e. both Conversational AI and Generative AI complementing each other to shape the customer experience landscape. A recent press release by Gartner, Inc. highlights the transformative potential of these technologies: By 2028, 60% of B2B seller work is projected to be executed through conversational user interfaces via Generative AI sales technologies, a significant leap from less than 5% in 2023.
By incorporating Generative AI models into chatbots and virtual assistants, businesses can offer more human-like and intelligent interactions. Conversational AI systems powered by Generative AI can understand and respond to natural language, provide personalized recommendations, and deliver memorable conversations.
Get ahead of the competition with Yellow.ai
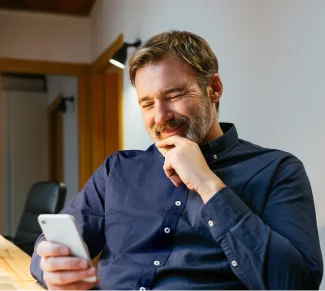
Frequently asked questions (FAQs)
Are Conversational AI and Generative AI used for different purposes?
Yes, they are. Conversational AI is primarily designed to facilitate human-like interactions, often used in chatbots, virtual assistants, and customer service tools to understand and respond to user queries in real-time. Generative AI, on the other hand, focuses on creating new content, whether it’s text, images, music, or other forms of data, by learning from existing patterns. While their core purposes differ, they can be integrated to enhance applications like chatbots, making them more dynamic and responsive.
How do generative AI models help in NLP?
Generative AI models play a pivotal role in Natural Language Processing (NLP) by enabling the generation of human-like text based on the patterns they’ve learned. They can craft coherent and contextually relevant sentences, making applications like chatbots, content generators, and virtual assistants more sophisticated. For instance, when a user poses a question to a chatbot, a generative AI model can craft a unique, context-aware response rather than relying on pre-defined answers.
What is the difference between deep learning AI and generative AI?
Deep learning is a subset of machine learning that uses neural networks with many layers (hence “deep”) to analyze various factors of data. It’s a technique that can be applied to various AI tasks, including image and speech recognition. Generative AI, on the other hand, specifically refers to AI models that can generate new content. While generative AI often uses deep learning techniques, especially in models like Generative Adversarial Networks (GANs), not all deep learning is generative. In essence, deep learning is a method, while generative AI is an application of that method among others.
What is the primary difference between Conversational AI and Generative AI?
At its core, Conversational AI is designed to facilitate interactions that mirror natural human conversations, primarily through understanding and processing human language. Generative AI, on the other hand, focuses on autonomously creating new content, such as text, images, or music, by learning patterns from existing data.
How are businesses currently leveraging these AI technologies?
Businesses are harnessing Conversational AI to power chatbots, virtual assistants, and customer service tools, enhancing user engagement and support. Generative AI is being employed in areas like content creation, design processes, and even product development, allowing for innovative solutions that often surpass human capabilities.
Are there any risks associated with implementing these AI technologies?
Like any technology, there are potential risks. The accuracy and effectiveness of AI models depend on the quality of data they’re trained on. Biased or incomplete data can lead to inaccurate or unfair outcomes. Additionally, over-reliance on AI without human oversight can sometimes lead to undesired results. It’s crucial for businesses to approach AI integration with a well-informed strategy and regular monitoring.
How do Generative AI and Conversational AI complement each other?
Generative AI can enhance the capabilities of Conversational AI systems by enabling them to craft more human-like, dynamic responses. When integrated, they can offer personalized recommendations, understand context better, and engage users in more meaningful interactions, elevating the overall user experience.
What’s the future outlook for these AI technologies?
The future is promising. With advancements in deep learning and neural networks, both Conversational and Generative AI are set to become more sophisticated and integrated into various sectors. As businesses recognize their potential, we can expect a surge in AI-driven solutions that cater to diverse needs, from customer support to creative content generation.