In a rush and need the TL/DR version?
Summarize
Generative AI reshapes business landscapes by creating new content through advanced machine learning. It offers significant benefits, from increasing productivity to enhancing customer interaction. Below are nine key advantages of generative AI for businesses:
- Creativity amplification: Generates diverse and engaging content autonomously.
- Time and cost savings: Automates processes, reducing the need for manual input and cutting costs.
- Hyper-personalization: Tailors customer experiences by analyzing individual data to personalize interactions.
- Enhanced efficiency and productivity: Optimizes business workflows, improving overall efficiency.
- Data synthesis: Analyzes and synthesizes large datasets to generate actionable insights.
- Realistic simulations: Provides accurate simulations for safer and more effective product testing.
- Adaptive learning: Continuously improves its outputs based on new data and feedback.
- Knowledge organization: Mines and categorizes information for easier access and discovery.
- Improved customer experience: Delivers more human-like, responsive customer service through AI.
Generative AI boosts efficiency and creativity and personalizes and enhances customer interactions, offering a significant competitive edge. Read the full blog for an in-depth exploration of these benefits and how platforms like Yellow.ai leverage generative AI.
Note: This summary is not a substitute for reading the article in full, as you may miss out on important nuances.
In today’s rapidly advancing world of artificial intelligence, a remarkable innovation known as generative AI has emerged, reshaping the very foundations of traditional rule-based systems. By harnessing the power of user data and preferences, generative AI goes above and beyond to provide personalized recommendations. This breakthrough technology empowers businesses to offer tailored solutions to their customers, elevating user satisfaction and engagement to unprecedented heights.
Let’s delve deeper into the world of generative AI as we explore its limitless possibilities. In this guide, you’ll learn what is generative AI and how you can harness its potential to propel your organization towards innovation and secure a coveted position at the forefront of this ever-evolving field.
What is Generative AI?
Generative AI, which falls under the umbrella of artificial intelligence, utilizes advanced deep learning models and large language models (LLMs) to generate top-notch content such as text, images, and more. These models tap into extensive training data to understand patterns and produce fresh and impressive outputs.
Venture capital firms have invested more than $1.7 billion in generative AI solutions over the last three years, with the most funding going to AI-enabled drug discovery and AI software coding.
From the latest research and advances in deep learning to practical generative AI examples and case studies of real-world applications, marketing, and media are already feeling the impacts of generative AI.
Gartner expects:
- By 2025, 30% of outbound marketing messages from large organizations will be synthetically generated, up from less than 2% in 2022.
- By 2030, a major blockbuster film will be released with 90% of the film generated by AI (from text to video), from 0% of such in 2022.

How does Generative AI work?
Generative AI models harness neural networks to analyze existing data and identify underlying patterns and structures, enabling them to generate fresh and innovative content.
One significant advancement in generative AI is the adoption of various learning approaches, such as unsupervised or semi-supervised learning during the training process. This enables organizations to effectively utilize large amounts of unlabeled data and create foundational models. These foundational models serve as a basis for AI systems that can perform multiple tasks.
Prominent examples of foundational models include GPT-3 and Stable Diffusion, which excel in language-related applications. For instance, ChatGPT, built upon GPT-3, enables users to generate essays based on concise text prompts. Conversely, Stable Diffusion empowers users to produce photorealistic images by providing text inputs.
By leveraging the power of generative AI models, organizations can tap into the potential of language and imagery, opening up exciting possibilities for content generation and creative applications.
Have heard about interactive Generative AI platforms like ChatGPT, Google Bard and Bing Chat, but don’t know the difference? Here is a breakdown of unique features and comparison of their capabilities. Read more.
A brief history of Generative AI
The field of generative AI continues to evolve rapidly, driven by ongoing research, advancements in deep learning techniques, and access to larger and more diverse datasets. As technologies progress, generative AI holds immense potential to revolutionize various industries and creative endeavors, unlocking new possibilities for content creation and human-machine collaboration.
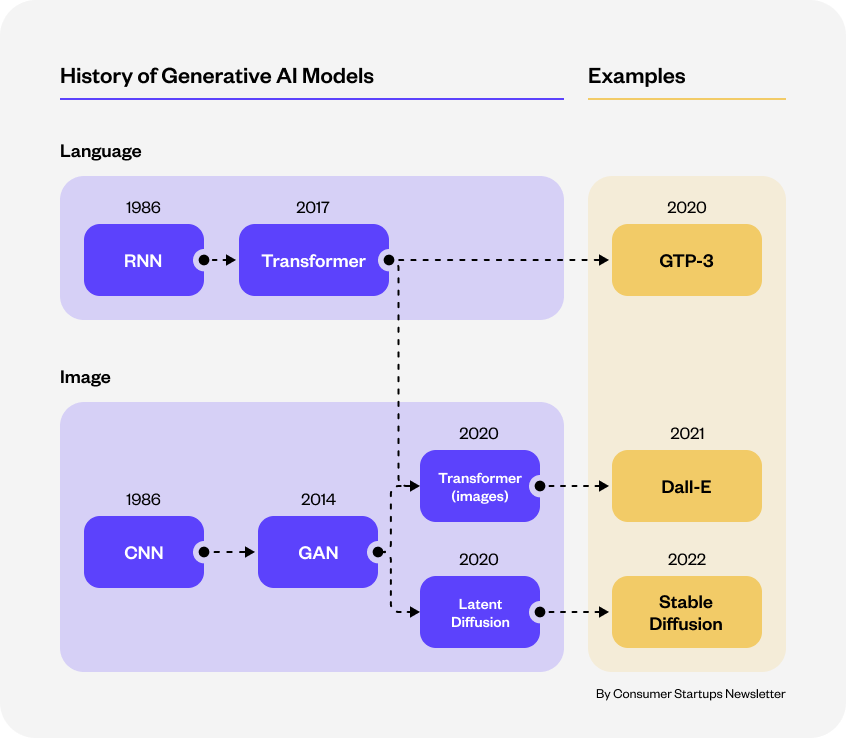
1. Early years (1950s-1970s):
The foundations of generative AI were laid during this period. Researchers began exploring the idea of machines capable of generating new content, such as music and art. Early attempts involved rule-based systems and expert systems.
2. Neural networks resurgence (1980s-1990s):
Neural networks experienced a resurgence, fueling advancements in generative AI. Researchers developed models like the Boltzmann machine and recurrent neural networks (RNNs), which demonstrated the ability to generate sequences of data.
3. Deep learning revolution (2010s):
Deep learning, enabled by the availability of large datasets and increased computational power, brought generative AI to new heights. Models such as variational autoencoders (VAEs) and deep belief networks (DBNs) pushed the boundaries of content generation and representation learning.
4. Adversarial networks (2014):
The introduction of generative adversarial networks (GANs) by Ian Goodfellow and his colleagues revolutionized the field. GANs consist of two components—a generator network that produces content and a discriminator network that evaluates the generated content for authenticity. GANs proved highly effective in generating realistic images, audio, and text.
5. Language models and text Generation (2010s-2020s):
Language models, particularly transformer-based architectures like OpenAI’s GPT (Generative Pre-trained Transformer), showcased remarkable text generation capabilities. Models like GPT-3 captured attention for their ability to generate coherent and contextually relevant text, leading to numerous creative applications.
6. Multi-modal and creative spplications (2020s onwards):
Recent advancements in generative AI have focused on multi-modal capabilities, combining text, images, and other modalities to generate rich and diverse content without pre-training or human interventions beyond prompts. AI-generated artwork, music, and virtual characters have garnered attention, demonstrating the potential of generative AI in creative domains.
What are the different types of Generative AI applications?
Generative AI has a wide range of potential applications, and its ability to generate new, realistic data has the potential to revolutionize many industries.
- Generative AI in NLP
- Generative AI in conversation design
- Generative AI in creating a knowledge base
- Generative AI to improve agent productivity
- Generative AI in omnichannel marketing
- Generative AI in customer lifecycle management
- Generative AI in Content Management
- Generative AI in intelligent segmentation
1. Generative AI in NLP
Generative AI for Conversational AI is one of the most exciting and rapidly developing areas of artificial intelligence. As AI continues to evolve, many generative AI companies have come ahead to harness the ability of generating human-like responses in a conversational setting. It has the potential to revolutionize the way we interact with machines, creating more natural and human-like conversations that are tailored to our individual needs and preferences.
Here are a few ways that generative AI can be used in automation platforms:
- NLP training data generation: Generative AI is used to generate new, realistic data that can be utilized to train machine learning models. This is particularly useful in situations where there is limited data available or when the existing data is biased or incomplete.
- Test data generation: Generate test data for software testing to save time and effort by eliminating the need for manually creating the test data. This is useful because it allows developers to test their software on a wide range of scenarios, which can help to uncover bugs and other issues.
- Process automation: Automate repetitive tasks such as data entry, document classification, and image recognition. This can help to improve efficiency and reduce errors.
- Decision support: Generate simulations and scenarios that can be used to support decision-making processes. For example, generative AI can be used to generate different production scenarios that can be used to optimize manufacturing processes.
2. Generative AI in conversation design
Currently, the problem with designing a conversational interface is that it’s important to take a bottom-up approach. This means designers need to envision what and how conversations will take place between users and chatbots and then design the conversation and its flow. This can be challenging as designers need to predict users’ inputs well ahead of time.
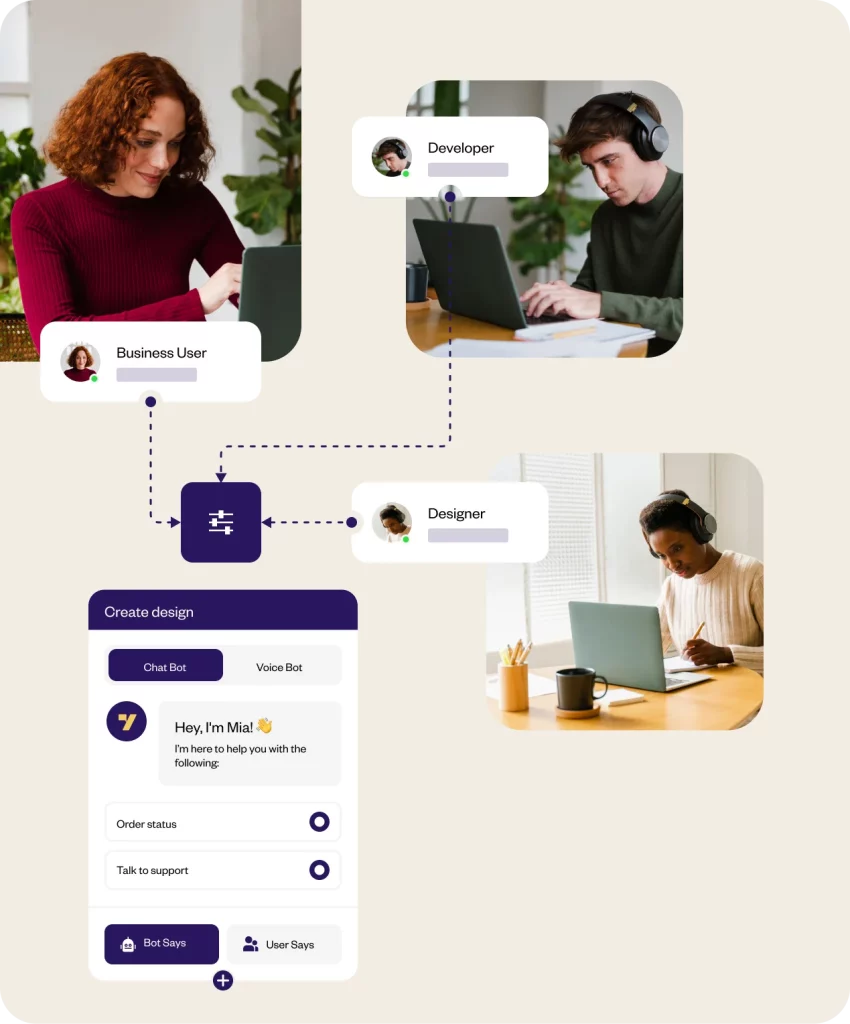
Generative AI can help in such cases while generating responses that enable designers to cover most of the user’s response as part of the conversation designs. Additionally, these models can be fine-tuned on specific datasets to improve their performance in a given domain.
Yellow.ai’s Dynamic Conversation Designer – a generative AI tool – allows you to design and launch conversations in minutes — no coding required! Try for free
Generative AI is becoming increasingly popular in the field of conversational design. From using traditional chatbots that rely on predefined scripts to respond to users, generative AI has created a revolution in getting trained on large datasets of human conversations to learn how to generate natural-sounding responses.
3. Generative AI in creating a knowledge base
Generative AI can be very helpful in creating a knowledge base by generating new content, summarizing existing content, categorizing content, and generating questions and answers.
- Content generation: Generative AI can be used to generate new text content, such as articles, blog posts, and product descriptions, that can be used to populate the knowledge base. This can help to ensure that the knowledge base is comprehensive and up-to-date.
- Summarization: Summarize existing text content, such as customer feedback and reviews, into shorter, more easily digestible formats. This can help to improve the overall accessibility and usefulness of the knowledge base.
- Categorization: Categorize content based on topics or keywords, which can make it easier for users to find the information they need. This can help to improve the overall usability of the knowledge base.
- Question and answer generation: Generate questions and answers based on existing content in the knowledge base. This can help to ensure that the knowledge base is addressing the most common customer inquiries and concerns.
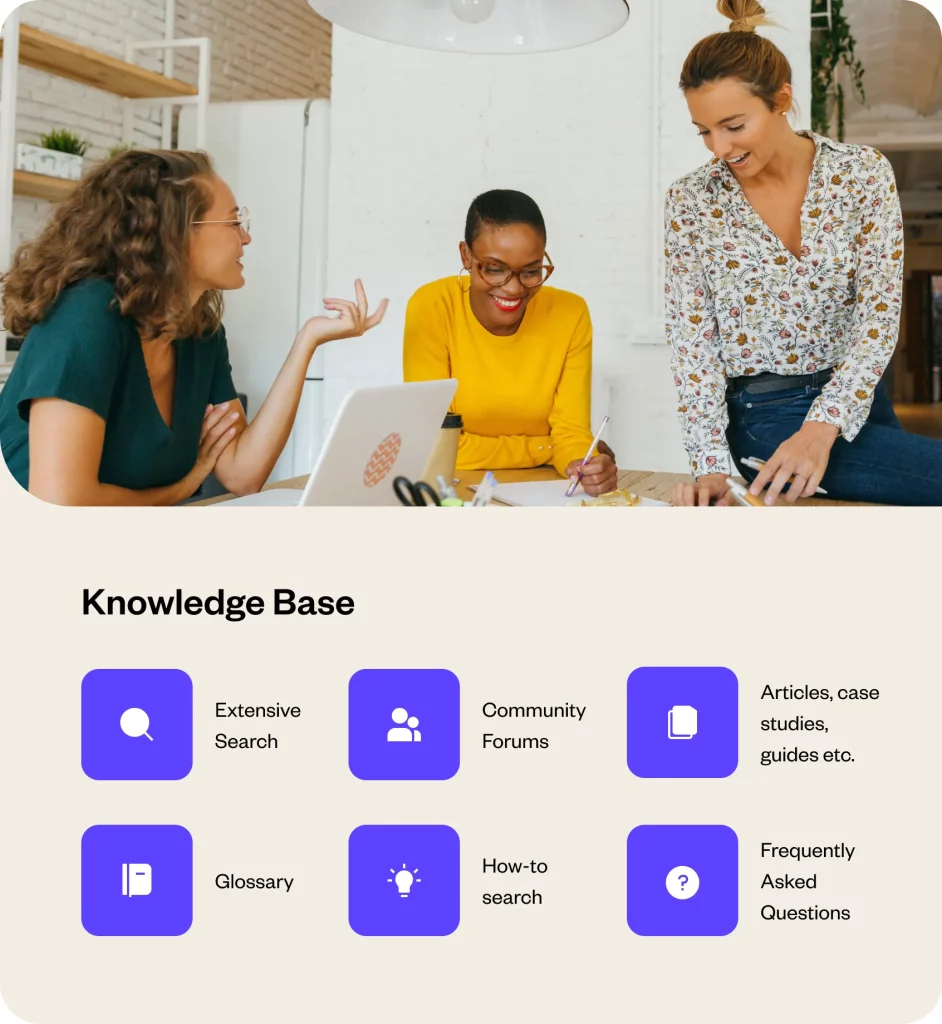
4. Generative AI to improve agent productivity
Generative AI improves agent productivity by automating routine tasks, providing automated responses to common inquiries, generating insights, and supporting sales activities. By using generative AI to support agents, organizations can improve overall customer experiences and increase efficiency. Few generative applications in customer service and support industry are –
- Chatbots and virtual assistants: Create chatbots and virtual assistants that can handle routine tasks and inquiries, freeing up agents to focus on more complex tasks that require human intervention.
- Customer insights: Analyze customer data and generate insights that can be used to improve customer experiences. This can help agents to better understand customer needs and preferences, which can improve the quality of customer interactions.
- Sales support: Generate personalized product recommendations and offers for customers, which can help to increase sales and improve customer satisfaction.
5. Generative AI in omnichannel marketing
Generative AI aids omnichannel marketing by generating personalized content and product recommendations that can be delivered across multiple channels.
- Personalized content: Generative AI can be used to create product descriptions, email subject lines, and social media posts, which can be tailored to individual customers based on their preferences and behavior. This can help to improve engagement and conversions across multiple channels.
- Product recommendations: Offer personalized product recommendations for customers based on their purchase history and behavior. These recommendations can be delivered across multiple channels, such as email, social media, and website pop-ups, to encourage customers to make additional purchases.
6. Generative AI in customer lifecycle management

By using generative AI to automate customer lifecycle management, organizations can improve customer retention, increase engagement, and drive sales.
- Onboarding: Generative AI can be used to create personalized onboarding experiences for new customers by generating welcome emails, personalized tutorials, and product recommendations based on their preferences and behavior.
- Customer engagement: Offer personalized content, such as product recommendations and blog articles, that can be delivered to customers at various stages of the customer lifecycle. This can help to improve engagement and increase the likelihood of repeat purchases.
- Customer service and support: Create chatbots and virtual assistants that can handle routine customer service inquiries, such as order status and product returns, freeing up agents to focus on more complex issues.
- Customer retention: Deliver personalized content, such as loyalty program rewards and targeted promotions, that can help to retain customers and encourage repeat purchases.
7. Generative AI in Content Management
- Content generation: Generative AI can be used to generate generate new articles, blog posts, and product descriptions, based on specific keywords or topics. This can help to ensure that the content is comprehensive and up-to-date.
- Content optimization: Optimize existing content by analyzing keywords, structure, and tone, and generating suggestions for improvement. This can help to improve the quality and relevance of the content.
- Translation: Translate content into multiple languages, which can help to reach a wider audience and improve accessibility.
- Metadata generation: Generate metadata, such as keywords, tags, and descriptions, which can help to improve search engine optimization (SEO) and make the content more discoverable.
- Content categorization and tagging: Generative AI can be used to categorize and tag content based on topics or keywords, which can make it easier to find and organize content within a content management system.
8. Generative AI in intelligent segmentation
- Customer behavior analysis: Analyze customer behavior, such as purchase history, website visits, and social media interactions, to identify patterns and generate insights that can be used to segment customers based on their behavior.
- Persona creation: Generative AI can be used to generate customer personas based on demographic, psychographic, and behavioral data. These personas can be used to segment customers based on their needs and preferences.
- Customized marketing campaigns: Generative AI can be used to fetch insights that can be used to create customized marketing campaigns for different customer segments. For example, generative AI can analyze customer data and generate insights that can be used to create targeted email campaigns, social media campaigns, and other marketing initiatives.
- Predictive modeling: Build predictive models that can be used to segment customers based on their likelihood to purchase, churn, or engage with a brand. These predictive models can be used to create targeted marketing campaigns and other initiatives.
What are Generative AI models?
Generative AI models are like creative machines that can produce new and original content in various formats on their own. These models are designed to understand patterns and structures in data and use that understanding to generate new data samples that resemble the original input. They learn from existing examples, like text, images, and music, and then use that knowledge to come up with their own original content. It’s kind of like teaching a computer to be artistic!
These models use advanced and complex algorithms and techniques to understand the patterns and relationships in the data they’ve been trained on. Once they’ve learned those patterns, they can generate new things that fit right in with what they’ve seen before.
Generative AI models have found applications in various domains, including natural language processing, image synthesis, music composition, and even video generation. They are instrumental in pushing the boundaries of creativity and enabling machines to generate original content that closely resembles human-generated output.
Types of Generative AI models
Generative AI encompasses various approaches and techniques for creating new content. Here are some of the prominent types of generative AI.
- Variational Autoencoders (VAEs)
- Generative Adversarial Networks (GANs)
1. Variational Autoencoders (VAEs)
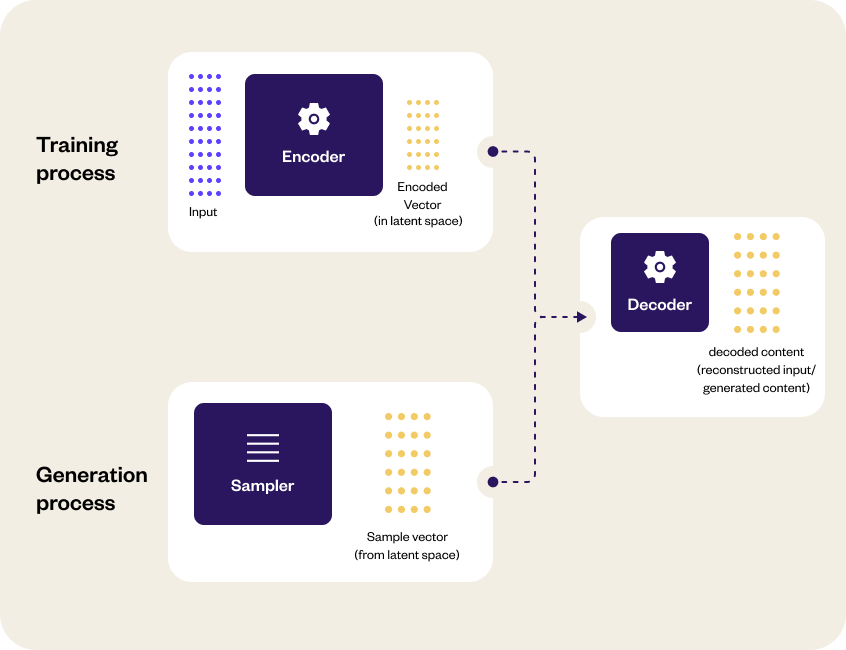
Variational Autoencoders (VAEs) are a type of generative AI model that combine concepts from both autoencoders and probabilistic modeling. They are powerful tools for learning representations of complex data and generating new samples.
At their core, VAEs consist of two interconnected neural networks: an encoder and a decoder. The encoder takes in an input data point and compresses it into a lower-dimensional representation called the latent space or latent variables. The encoder network captures the underlying structure and patterns of the input data, mapping it to a latent space that follows a specific probability distribution.
The decoder network then takes a sample from the latent space and reconstructs it back into the original input space. The decoder learns to generate new samples by learning the mapping from the latent space to the input space. This allows VAEs to generate new content by sampling from the learned distribution in the latent space and decoding it into meaningful outputs.
VAEs have found applications in various domains, including image generation, text generation, and data compression. They enable the synthesis of novel content by learning meaningful representations of the input data and leveraging the power of probabilistic modeling. Through VAEs, machines can generate new and original content that captures the essence and patterns of the data they were trained on.
2. Generative Adversarial Networks (GANs)
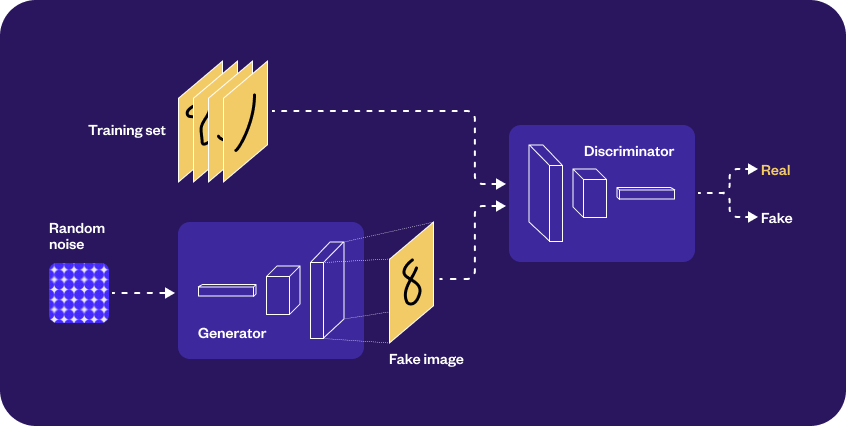
Generative Adversarial Networks (GANs) are a class of generative AI models that have revolutionized the field of artificial intelligence by generating realistic and high-quality content. GANs consist of two neural networks: a generator and a discriminator, which work in a competitive setting.
The generator network takes random noise as input and generates synthetic samples, such as images, based on that noise. Initially, the generator produces crude outputs that do not resemble the desired data distribution. The discriminator network, on the other hand, receives both real and generated samples and aims to distinguish between them accurately. It learns to differentiate between real and fake samples by updating its weights during the training process.
GANs have demonstrated remarkable success in generating high-fidelity images, realistic audio, and even text. They have been employed in various creative applications, such as art generation, image synthesis, and data augmentation. GANs have also found applications in improving image quality, anomaly detection, and style transfer.
Top 7 generative AI examples by industry
1. Generative AI in advertising and marketing
Generative AI is revolutionizing the field of advertising and marketing, providing innovative solutions to enhance campaign effectiveness and customer engagement. It can analyze customer data, preferences, and behavior to create highly personalized and targeted marketing content. This includes dynamic advertisements, personalized product recommendations, and customized email campaigns. By delivering tailored content to individual customers, generative AI enhances engagement and conversion rates.
2. Generative AI in finance
Generative AI algorithms can analyze vast amounts of financial data to detect patterns and anomalies that indicate fraudulent activities. By continuously learning from new data, these models improve their accuracy over time, enabling financial institutions to proactively identify and prevent fraudulent transactions, safeguarding the integrity of their systems, and protecting customers’ assets. It also plays a crucial role in algorithmic trading by analyzing vast amounts of market data and identifying profitable trading opportunities in real time. These models can execute trades at high speeds, leveraging advanced algorithms to capitalize on market inefficiencies and optimize trading strategies, potentially leading to improved profitability.
3. Generative AI in healthcare
Generative AI is revolutionizing healthcare by enabling advancements in medical imaging, drug discovery, disease prediction, data augmentation, medical text generation, and personalized treatment planning. It enhances imaging techniques, accelerates drug development, predicts disease onset, generates synthetic medical data, automates documentation, and tailors treatments to individuals. However, ethical and regulatory considerations must be addressed to ensure responsible implementation. Generative AI has the potential to transform healthcare by improving diagnostics, treatment, and patient care.
4. Generative AI in manufacturing
Generative AI has brought about a remarkable revolution in the world of Manufacturing and Production. Manufacturers now have access to an endless array of data sources, allowing them to gather valuable insights. This data plays a crucial role in their day-to-day decision-making, whether it’s derived from traditional sensor devices, real-time video streams, or even manually compiled reports. It goes beyond simply enabling impressive design capabilities; it empowers engineers to delve into massive data sets, aiming to enhance safety measures, create simulation datasets, explore faster manufacturing or machining methods for parts, and expedite the overall product launch process.
5. Generative AI in agriculture
Generative AI has emerged as a game-changer for the agri-tech sector, revolutionizing agriculture by addressing pressing challenges such as climate change, food security, and population growth. With its transformative capabilities, this advanced technology offers a multitude of benefits that drive sustainable growth in the agricultural industry.
One of the significant advantages of integrating generative AI in agriculture is the application of predictive analytics. By leveraging vast datasets encompassing crucial factors like weather patterns, soil conditions, and crop health data, AI models can accurately forecast future outcomes. This valuable insight empowers farmers to make informed decisions, leading to increased crop yields and enhanced profitability.
6. Generative AI in education
Generative AI is transforming education by enabling personalized learning experiences, adaptive tutoring, and intelligent content creation. It analyzes student data to provide personalized feedback and guidance, recommends tailored educational resources, and facilitates language learning and translation. Generative AI also empowers educators with learning analytics and automated assessment, while virtual reality and augmented reality technologies enhance immersive learning. This technology is revolutionizing education, promoting individualized learning, and fostering innovation in the digital age.
7. Generative AI in cybersecurity
Generative AI is revolutionizing cybersecurity by detecting threats, analyzing malware, assessing vulnerabilities, enhancing user authentication, and providing realistic training simulations. This technology strengthens defenses against evolving cyber threats, safeguarding data and systems. Generative AI’s advanced algorithms enable real-time threat detection and proactive response, minimizing potential risks. By automating patch management and authentication processes, it enhances overall cybersecurity posture, ensuring robust protection against cyberattacks.
Generative AI vs Conversational AI
Generative AI and Conversational AI are two key components driving advancements in customer experience. Generative AI focuses on creating original content and generating human-like responses, while Conversational AI aims to facilitate natural and engaging interactions with users. By combining these technologies, businesses can provide highly personalized and contextually relevant experiences to their customers. The synergy between Generative AI and Conversational AI opens up never-before-seen possibilities, enabling virtual assistants and chatbots to deliver more authentic, intelligent, and satisfying customer experiences.
Related read: Chatbots vs Conversational AI – What’s the Difference?
Aspects | Generative AI | Conversational AI |
Focus | Generative AI focuses on creating new content based on existing data patterns and examples. | Conversational AI focuses on enabling human-like conversations between machines and humans. |
Application | Generative AI is used for creative tasks such as content creation, personalized marketing, and design generation. | Conversational AI is applied in chatbots, virtual assistants, voice assistants, and customer service automation. |
Personalization | Generative AI enables personalized content creation, allowing tailored recommendations and dynamic advertisements. | Conversational AI enhances personalization by providing real-time, context-aware assistance based on user queries and preferences. |
Engagement | Generative AI-powered content can captivate customers with visually appealing designs and interactive experiences. | Conversational AI engages customers through interactive conversations, providing immediate responses and fostering a sense of connection. |
Convenience and efficiency | Generative AI streamlines creative processes and content generation but does not directly contribute to convenience and efficiency. | Conversational AI offers self-service options and automates routine tasks, providing instant support and saving time for customers. |
Enhanced support | Generative AI does not directly contribute to enhanced support. | Conversational AI systems handle a large volume of customer inquiries simultaneously, providing 24/7 support and resolving common issues. |
Integration | Generative AI-generated content can be integrated into conversational interfaces, creating consistent branding and messaging. | Conversational AI can be seamlessly integrated into various platforms and touchpoints, ensuring a coherent customer experience. |
Create AI bots in seconds using generative AI powered YellowG
See how
Benefits of leveraging generative AI with Yellow.ai

At Yellow.ai, we use a combination of generative AI technology and our Dynamic Automation Platform (DAP ), which holds all the data, behavior, and experiences collected by our Dynamic AI agents. Using this, we aim to deliver improved business results across the breadth of use cases including automation, agent support, and marketing conversations. Businesses will get benefits such as –
Related read: 9 Benefits of generative AI in enterprises
1. Reduced costs and improved efficiency in bot building
According to a survey conducted by Deloitte, set-up challenges, including training data and maintenance, were among the top reasons for not implementing chatbots in enterprises. While enterprises have many documents with product and support information, barely 25% of that is captured in manually configured QnA bots.
The solution offered by Yellow.ai:
Generative AI in bot building

- Generative AI in Yellow.ai will help the chatbot developers by suggesting the best next step in conversational flow automation so that all the scenarios are covered and maximum automation is achieved. Also, it anticipates how users might respond to the chatbot and then design user journeys based on set goals.
- With the help of generative AI, testing data is generated automatically so the user avoids having to write it one by one and will be able to bulk test the generated utterances. Auto-generating test data helps in reducing the testing time by 50%.
- The main aim of Document Cognition, Yellow.ai’s knowledge discovery solution, is to help users get instant and accurate answers to their queries from unstructured formats (data and images) using NLP and Machine Comprehension. Yellow.ai Document Cognition and keywords also search content based on the subject and meaning. Let’s say – the keyword is “credit limit” and the user asks “What is the highest amount that can be sanctioned?” Document Cognition can respond to the user with the right answer to this contextually related question.
Benefits:
- Faster time to market – Go live within minutes by automating FAQs from documents/URL
- Better coverage of FAQs – Train your bot on thousands of FAQs from multiple sources
- Improved accuracy – Achieve more than 97% intent accuracy from day one
2. Enhanced customer engagement
Successful customer engagement requires a customer-centric approach, effective communication, and a willingness to adapt to changing customer needs and preferences.
Customers today expect a seamless and consistent experience with a personal touch, and any deviation from this can be a turn-off. Many enterprises struggle to customize their interactions with customers, which can result in disengagement or even frustration.

The solution offered by Yellow.ai:
Generative AI in marketing campaigns
- Organizations can improve engagement rates & reduce drop-offs along a buyer’s journey by using contextual nudges across channels including web, text, social, voice, etc.
- Based on parameters such as audience type, user behavior and the campaign goal, the AI-powered template manager generates the most relevant content for a marketing campaign. Marketers can nurture leads, and interact with users via these conversations to understand their preferences and purchase intent.
Benefits:
- Improve lead generation by 20% – Generate high-quality leads with accurate targeting
- Increase customer engagement by 60% – Nudge users at the right time, on the right channel
- Improve CSAT by 40% – Get contextual history across different channels and communicate effectively
3. Improving agent productivity
The quality of customer service is a major factor in determining CSAT scores. Enterprises that provide high-quality customer service, such as responsive and friendly support, are more likely to receive positive feedback from customers. However, businesses of all sizes face common problems, such as:
- 30% of agents spend considerable time crafting repetitive responses to communicate to customers rather than looking for solutions to help them.
- More than a fifth of the costs of running a contact center are incurred in coaching agents and ensuring the quality of support.
- Contact centers grapple with high attrition rates. Managing support quality becomes a huge challenge given the agent turnover.

The solution offered by Yellow.ai:
Generative AI in customer service
- Agents can get suggested replies to customers’ queries with the help of Generative AI in Yellow. This will help agents to understand customer issues faster since they don’t have to read through current or past ticket transcripts. Also, agents can view the timeline of recent tickets and summaries of any specific ticket to provide quicker first response to customers and faster resolution, eventually leading to better CSAT.
- Based on agents’ activities, with the help of generative AI in Yellow, the platform creates coaching insights for agents that will help them improve their performance and productivity.
Benefits:
- Improved “First response time (FRT)” and “First contact resolution rate (FCR)”
- Improved agent productivity and CSAT
- Save cost and time spent on quality assurance controls and improve support quality
Generative AI can be used in many ways to enhance customer experience. From training your chatbots to interact with customers and providing them with quick and accurate responses to their queries to helping your customers with various tasks, such as booking appointments, ordering products, or finding information — generative AI can help businesses provide a more personalized and efficient customer experience, leading to increased customer satisfaction and loyalty.
Using Yellow.ai’s Generative AI platform, create chatbots and virtual assistants that can handle complex conversations. By using our powerful platform, you can automate customer support, lead generation, and other business processes to improve efficiency and provide a better customer experience.
See generative AI in action
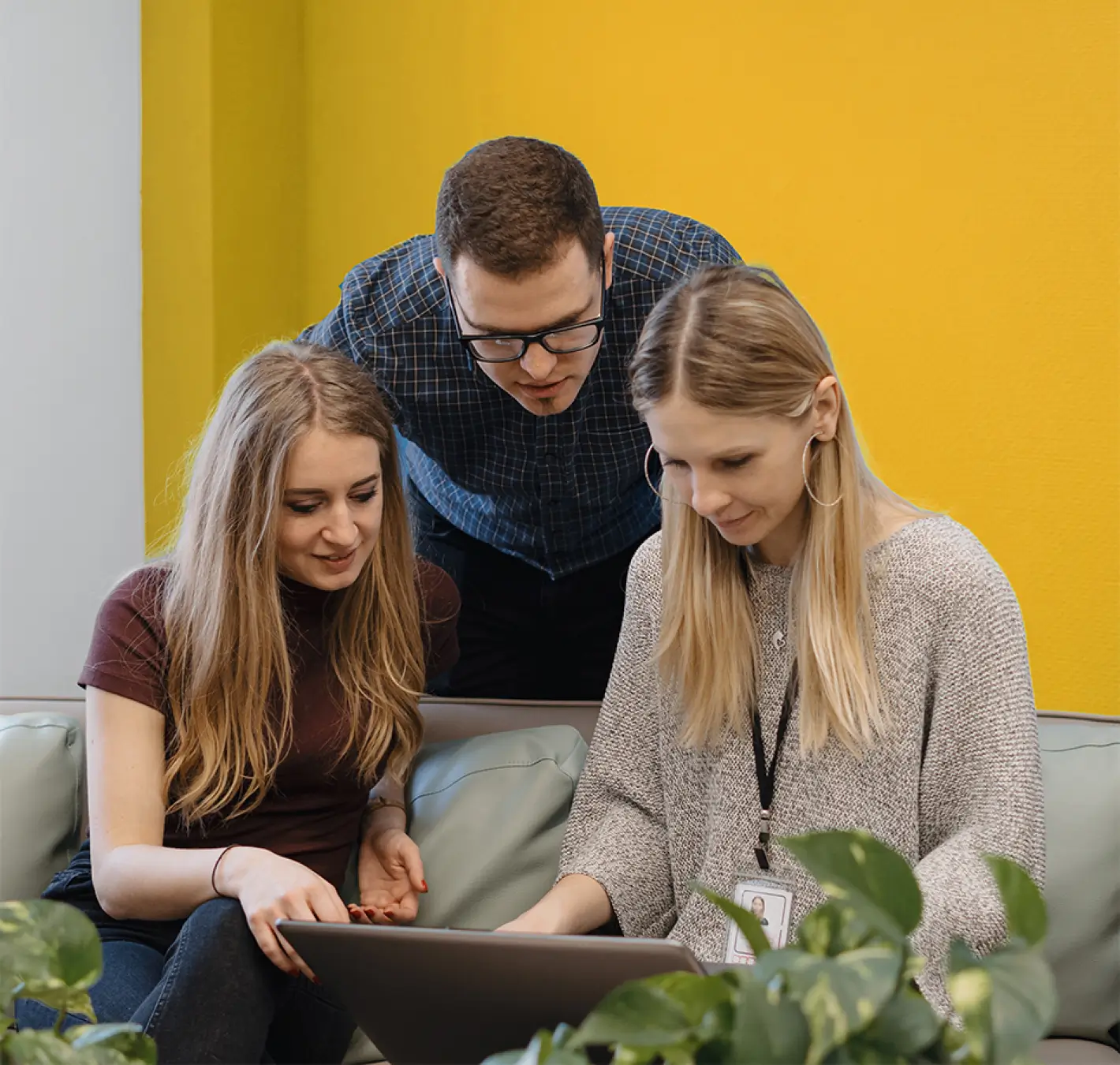
Key considerations for enterprises for Generative AI-powered conversational systems
Generative AI enhances customer engagement by enabling dynamic AI agents with human-like responses in conversational AI systems. It creates personalized content, streamlines conversational flows, and optimizes conversational marketing campaigns.
Related read: Goal-based conversations, powered by generative AI
Generative AI improves customer support through ticket summarization, assists in designing conversational flows, and facilitates goal-based marketing campaigns. Enterprises should define objectives, partner with automation experts, and prioritize ethical considerations for successful implementation. By leveraging generative AI, enterprises elevate customer experiences with personalized, natural-language interactions and stay ahead in customer engagement trends.
What Enterprises Should Know About Generative AI-Powered Conversational Systems
Potential pitfalls of Generative AI
While generative AI has many benefits, there are also concerns that businesses and individuals should be aware of. These include:
- Misinformation: Generative AI can be used to generate fake or misleading content, such as deep fakes, which can be used to spread misinformation or propaganda.
- Data privacy: Businesses require large amounts of data to be trained, and this data can include personal information. Businesses and individuals need to ensure that they are collecting and using data ethically and in compliance with data privacy regulations.
- Security: Generative AI can be vulnerable to cyber-attacks and other security threats, which can result in the theft or misuse of sensitive data.
- Ethical concerns: The use of generative AI can raise ethical concerns around the responsibility of businesses and individuals in ensuring that it is used ethically and in a way that aligns with social and cultural values.
Businesses and individuals need to be aware of these concerns and take appropriate steps to address them, such as ensuring that generative AI models are trained on unbiased data, using it responsibly and ethically, and implementing strong security measures to protect sensitive data.
Best Generative AI applications and tools
Other then Generative AI-powered conversational AI platforms like Yellow.ai, there new several generative AI applications and tools that offer diverse capabilities to businesses and developers, enabling them to leverage the power of AI in various domains. Whether it’s creating engaging content, generating code, writing poetry, or producing dynamic videos, these tools can enhance productivity and user experiences.
- GPT-4: The latest version of OpenAI’s language model, GPT-4, is designed to understand and generate text more effectively, making it great for creating engaging content and generating natural-sounding responses.
- ChatGPT: Developed by OpenAI, ChatGPT allows for interactive and dynamic conversations with users. It’s like having a chat with an AI assistant that can provide realistic and engaging responses.
- AlphaCode: AlphaCode is a powerful tool that focuses on understanding and generating natural language. It can be used to develop advanced conversational experiences that feel more human-like.
- GitHub Copilot: If you’re a developer, GitHub Copilot can be a helpful companion. It uses generative AI to suggest code snippets and assist with coding tasks, making your development process smoother and more efficient.
- Bard: Bard is an AI model developed by OpenAI that specializes in generating poetry. It can create beautiful and coherent poems, showcasing the artistic side of generative AI.
- Cohere Generate: Cohere Generate is a versatile tool that can generate human-like text based on prompts. It can be used for various applications such as creating content, generating chatbot responses, and more.
- Claude: If you need assistance with writing, Claude can be your writing companion. It uses generative AI to provide real-time suggestions, correct grammar, and improve writing style, helping you write better and more effectively.
- Synthesia: Synthesia is a video generation platform that uses generative AI to create personalized and dynamic videos. It allows businesses to generate customized video content at scale, enhancing their marketing and communication efforts.
- DALL-E 2: DALL-E 2 is an advanced generative AI model that can generate high-quality images based on textual descriptions. It opens up exciting possibilities for creative image synthesis and visual content generation.
Generative AI – Frequently asked questions (FAQ)
How does generative AI work?
Generative AI utilizes machine learning algorithms to generate new data by recognizing patterns in existing data. It involves training models on large datasets and enabling them to generate new content, such as text, images, or even videos. The models learn the underlying patterns and characteristics of the data and use that knowledge to create new, unique outputs.
What is the benefit of generative AI?
Generative AI offers infinite possibilities. It allows for the generation of new and creative content, which can be useful in various fields such as art, marketing, design, and many others. It also helps in automating tasks that require content creation, saving time and resources. Generative AI can also enhance personalization by generating tailored recommendations or responses based on user preferences and behavior.
What is the difference between generative AI and cognitive AI?
Generative AI focuses on generating new content or data based on existing patterns, while cognitive AI aims to simulate human intelligence by understanding, reasoning, and learning from data. While generative AI is primarily concerned with content creation, cognitive AI involves broader capabilities like natural language understanding, problem-solving, and decision-making.
What is the difference between generative AI and NLP?
Generative AI and Natural Language Processing (NLP) are related but distinct concepts. Generative AI refers to the ability to generate new content or data, including text, while NLP focuses on understanding and processing human language. NLP encompasses tasks like text classification, sentiment analysis, and language translation. Generative AI can be a component of NLP systems, where it generates text or helps in text generation tasks.
How is generative AI being used today?
Since Generative AI is still in it’s nascent stages, new powerful use cases are being discovered every day. As of today, generative AI already finds powerful applications in various fields. It is used in content generation, creative arts, virtual assistants, chatbots, and personalized recommendations. It can generate realistic images, mimic human speech, and automate content creation processes. Generative AI is also utilized in data augmentation, where it generates synthetic data to expand training datasets for machine learning models.
What are the most interesting uses of generative AI?
Generative AI has unlocked a multitude of captivating and groundbreaking applications across various domains. One fascinating use is in content generation, where generative AI models can autonomously produce text, images, and videos. This capability has the potential to revolutionize content creation, enabling the automatic generation of articles, stories, artwork, and even virtual characters. With generative AI, the boundaries of creativity and automation can be pushed further, offering exciting opportunities for industries such as marketing, entertainment, and media.
Another intriguing application of generative AI lies in image synthesis and editing. Advanced models, like generative adversarial networks (GANs), can synthesize realistic images or manipulate existing ones. This technology finds applications in gaming, where lifelike visuals are essential, as well as in image editing tools that leverage generative AI for tasks like style transfer, super-resolution, and object manipulation. By empowering users to create and modify images with impressive realism and precision, generative AI expands the possibilities of visual content creation and manipulation.
These are just a few examples of the diverse and exciting applications of generative AI. As the technology continues to evolve, we can expect even more innovative and transformative uses in the future.
What are some examples of generative AI?
Generative AI encompasses several remarkable examples that showcase its capabilities across various domains. OpenAI’s GPT is a renowned model for generating human-like text responses, while DALL·E demonstrates the generation of images based on textual prompts. DeepArt combines deep learning with artistic styles to transform photos into unique artwork. Google’s Magenta project focuses on generative AI for music and art, enabling users to create original compositions. NVIDIA’s StyleGAN excels at generating realistic and high-quality images. These examples highlight the vast potential of generative AI in content creation, image synthesis, music composition, and artistic expression. The advancements in generative AI models continue to push the boundaries of creativity and pave the way for innovative applications in various fields.
How is generative AI used in consulting?
Generative AI can be utilized in consulting to automate and enhance various tasks. It can assist in generating reports, analyzing data, providing recommendations, and simulating scenarios. For example, generative AI models can help generate realistic financial forecasts, simulate market scenarios, or provide personalized recommendations for clients based on their specific needs and goals.
What are the limitations of generative AI?
Generative AI has some limitations. Generating high-quality content requires large and diverse training datasets. It may struggle with generating coherent and contextually accurate outputs. Generative AI models can also be prone to biases present in the training data. Another challenge is the interpretability of the generated results, as it can be difficult to understand and explain the decision-making process of the models.
Does generative AI use deep learning?
Yes, generative AI often uses deep learning techniques, such as deep neural networks. Deep learning models, like generative adversarial networks (GANs) and recurrent neural networks (RNNs), are commonly employed in generative AI tasks. These models can capture complex patterns and dependencies in the data, allowing for the generation of more sophisticated and realistic outputs.
What is the impact of generative AI?
Generative AI has a significant impact across various industries. It enhances automation, creativity, and personalization. It enables the generation of realistic and engaging content, improves user experiences, and increases productivity by automating content creation processes. Generative AI also has the potential to transform industries like entertainment, marketing, design, and healthcare, opening up new possibilities for innovation and advancement.
Is generative AI replacing human creativity?
No, generative AI is not replacing human creativity. Instead, it augments human creativity and provides new tools for expression and innovation. Generative AI can automate certain creative tasks, generate ideas, and inspire human creators. However, human creativity remains unique and irreplaceable, as it involves complex emotions, experiences, and subjective perspectives that AI cannot fully replicate. Generative AI serves as a powerful tool that complements and collaborates with human creativity to take it several notches higher rather than replacing it.