In this struggling economy, businesses are seeking ways to help them maximize ROI and reduce costs. The answer to this is Conversational AI to automate the interactions with customers, all the while reducing manual efforts.
However, a significant obstacle faced by businesses in adopting these virtual assistants is out-of-context or false responses from the bots. Technically this challenge is known as out-of-distribution (OOD). Detecting and handling such OOD responses is crucial for improving user interactions, providing correct information, ensuring system robustness, and enabling generalization across industries.
We recently published a paper, “BED: Bi-Encoder-Based Detectors for Out-of-Distribution Detection,” in which we deep-dive into the concept of out-of-distribution (OOD) responses with a proposed method called BED (bi-encoder-based detectors) for OOD detection within conversational AI platforms, ensuring the accuracy and reliability of the bot responses.
For more detailed information on our proposed method and experimental results, refer to our research paper, “BED: Bi-Encoder-Based Detectors for Out-of-Distribution Detection.”
Understanding Out-of-Distribution (OOD)
OOD responses occur when the conversational AI model generates answers that do not align with the topic or context of the conversation. These responses may seem random, irrelevant, or unrelated to the user’s queries.
Let’s take a sample bot conversation to understand this.

In this example, the bot’s mention of pancakes instead of health insurance showcases an OOD response. Ideally, a bot should stick to the domain, in this case, “health insurance,” so that a relevant and accurate response is provided to users.
Read more: Generative AI – The ultimate guide for 2023
What is OOD detection and its importance
OOD detection is the process of identifying instances that differ significantly from the training data distribution. These instances are often considered novel, unseen, or anomalous examples. OOD detection finds applications in various fields, including natural language processing, computer vision, and anomaly detection. In natural language processing, OOD detection can identify user inputs outside the expected range of queries or topics.
OOD detection is super critical as it prevents unreliable predictions or erroneous decisions when machine learning models encounter data points that fall outside their training data.
It helps in filtering out irrelevant or potentially harmful input, enhancing the reliability and safety of conversational AI systems.
Additionally, OOD detection helps in the generalization and adaptation of NLP models. By recognizing when a new input does not belong to its dedicated distribution, models can adapt their behavior based on this detection. This allows for better handling of unseen or novel data, improving the model’s ability to handle diverse inputs effectively.
Introducing BED: Bi-encoder-based detectors for OOD detection
Bi-encoders are widely used in Natural Language Processing (NLP) tasks like sentence similarity and text classification. BED is a new method based on bi-encoder-based detectors that helps with OOD detection. It leverages bi-encoders’ power to extract meaningful representations from textual data, enabling efficient detection mechanisms.
BED helps to achieve superior OOD detection even without labeling the OOD samples during training, thereby simplifying the process of NLP training.
BED helps to achieve near 0% hallucinations. It allows the system to flag inputs that fall outside the distribution of its training data, thereby preventing the generation of unreliable or fabricated responses.
Commenting on the BED, Jaya Kishore Reddy Gollareddy, co-founder and CTO of Yellow.ai, said, “Based on our benchmarking results, BED succeeds in outperforming other detectors that also don’t require OOD samples in the training data. In some cases, they even outperform detectors that utilize OOD samples in training. When OOD samples are absent in the training data, bi-encoder-based detectors perform better than other methods across all datasets.”
Benefits of BED in conversational AI
Implementing BED in conversational AI systems offers several advantages:
Near 0% hallucinations: Firstly, it helps detect a significant percentage of OOD responses, reducing the likelihood of irrelevant or undesirable bot behavior. When an OOD response from the bot is detected, fallback strategies like transferring the user to a live agent or seeking clarification can ensure a seamless user experience.
Enhance user experience: OOD detection helps enhance the user experience in conversational AI products. By detecting when a user input falls outside the expected scope of the system, it enables the AI to provide appropriate responses. This prevents the system from generating irrelevant or misleading replies and ensures a more satisfying and meaningful conversation for users.
Genuine transfer to human agents: BED intelligently differentiates between queries that can be handled by bots and those that require the involvement of human agents. This smart filtering ensures that human agents are utilized for valuable queries that genuinely require their intervention, resulting in improved efficiency and deflection rates.
Conclusion
By accurately identifying the responses that are not part of NLP training data, BED prevents OOD, thereby enhancing the robustness, safety, and reliability of the Yellow.ai platform. BED prevents hallucinations and ensures accurate responses to improve the user experience.
Experience Enterprise-grade Generative AI
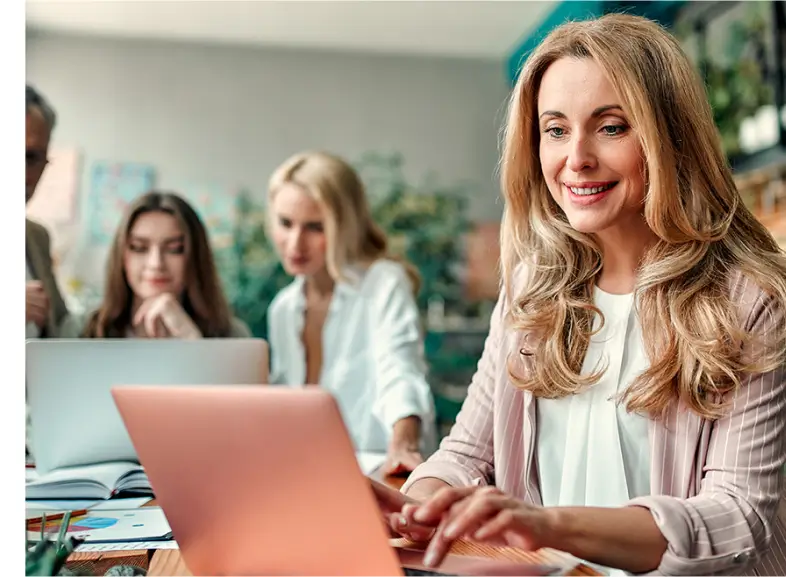